10 Key Aspects of Data-Driven Business Intelligence
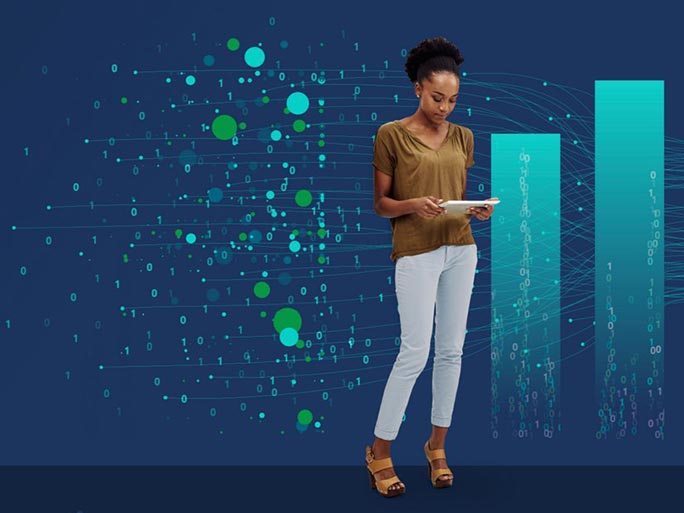
Qlik outlines 10 areas that technology leaders will need to consider to get the most out of their data strategy.
Today, both businesses and society at large are immersed in an environment governed by uncertainty and caution. Geopolitical and economic problems have led to an increasing tendency toward isolation.
Meanwhile, conflicts and local regulations are multiplying, with the consequent need to adapt to new legal frameworks. On the economic front, mistrust reigns, with fears of recession, rising interest rates, and inflation affecting lending and investment.
More than a few experts claim that we are in a process of de-globalization. As a result, there is no other solution than to adapt to it in order to be able to react to it and foresee what is to come in the near future. In this context, Qlik has identified 10 areas that technology leaders need to focus on to make the most of their data initiatives and achieve success:
1 – Real-time data
In today’s environment, there is a need to update your supply chains from batch to near real-time data. As more perimeter devices emerge on the network that produces a high volume of continuous information streams, more opportunities to leverage real-time data will emerge and companies must capitalize on these efforts. For this reason, real-time data helps combat supply chain disruptions.
2 – Speed of decision
The next step is to adapt operational decisions at the same pace. Analytics, AI, and automation can make more decisions than humans and do it faster, but the role of people is still key in aspects such as digital hygiene or information filtering. The speed of decision-making at scale makes it possible to shorten the process between data and action.
3 – Optimising development
With the emergence of low-level programming tools for creating applications, non-technical users develop their own apps, drive the creation of new software and increase the consumption of data and knowledge. In this way, optimization of app development can be achieved with both low and high-level programming, and organizations should not have to choose between low and high-level programming, but the key is to go for optimization.
4 – Human-machine relationship
By now, natural language models have been trained on huge datasets, causing some models such as GPT-3 to open the debate about whether machines will finally pass the Turing Test. Natural language will have huge implications for the way we query, interpret, and report on information in the data and analytics space.
5 – Data that moves to action
A decade-long mantra in the data industry is to provide the right information to the right user at the right time. In this case, the most useful model for connecting the user to the action is data storytelling, a way of delivering information in a way that the receiver understands and can interpret it without the need for an actor to act as an interpreter.
6 – New opportunities
In a context that tends towards fragmentation, there is also a market trend in the opposite direction: convergence. Systems that were once siloed are now consolidating, including the integration of data, management, analytics, AI, visualization and more. Combining these functions opens the door to previously unimaginable opportunities for interoperability.
7 – Modernisation in the cloud
During the pandemic, enterprises modernized their applications and migrated data to the cloud. This has led to the emergence of venture capital-driven start-ups, each specializing in one area. Many of these will disappear as sectors mature and consolidate, and the market will experience a huge wave of mergers and acquisitions as smaller providers start to look for an exit.
8 – Fabric X
Data fabric has recently come to the fore as an important methodology that connects distributed datasets through semantic modeling. In this environment, other fabrics or “X-tissues” are needed, including the application fabric, the BI fabric, or the algorithmic fabric.
9 – Deeper AI
AI is integrated at a deeper level of the process. In the process, they are being ‘cross-pollinated’, generating new insights that were previously unthinkable. The use of AI in data management makes it possible to automate more of the routine tasks of data engineering.
10 – Derived and synthetic data
Data is a liquid asset as it can be viewed differently for different purposes. Data that is transformed, processed, aggregated, correlated, or used to execute operations is called ‘derived’ data. Synthetic data is data that has not been derived from actual trades. Increasing its use is critical for decision-making.