Agentic AI: a New Step on the AI Pathway
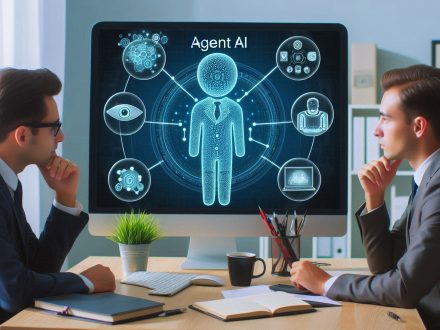
The development of agentic AI is one of the most important technological trends in 2025. What is it, what are its benefits, and what are its main applications?
Generative AI has been the technology that has generated the most buzz since OpenAI launched ChatGPT to the public at the end of November 2022.
In the last two years, the growth of this technology has been spectacular, with new use cases being discovered almost every day. But now it is the turn of agentic AI or autonomous agents.
At the end of last year, in our report on the trends that will shape the technology sector in 2025, we highlighted the development of agentic AI. ‘This will be the year we start to see the hype around agentic systems begin to bear fruit,’ Snowflake said.
‘In 2025, AI agents will be king. According to internal Salesforce data, up to 92% of developers believe that AI agents will positively transform their careers,’ specified Salesforce.
What is Agentic AI?
Salesforce explains that ‘agentic AI represents the next evolution of automation’. ‘Unlike traditional AI chatbots, which require manual instructions or rigid programming, this new agent AI responds dynamically to real-time data and evolving business needs,’ it says.
‘Agentic AI consists of intelligent systems that have greater capacity and autonomy for perception (information interpretation), reasoning and decision-making, just as a human would. It focuses on putting to work, in orchestration, different AI agents that together can create results more closely linked to the cognitive capacity of humans. This is known as multi-agent AI systems. This brings multiple benefits at all levels: cost reduction, search for efficiencies in the value chain, improvement in customer service, reduction of time spent on an activity, etc.,’ says Fran Monzonis, Chief AI Officer at Capgemini.
Thus, Rudy Galfi, Director of Product Management at Celonis, says that ‘agentic AI represents a major shift from AI systems that responded passively to cues to systems that can autonomously pursue goals, reason through complex tasks, and interact with systems and data in dynamic environments’. He further highlights the ‘increasing ability of AI agents to operate in multiple situations, make context-aware decisions and self-correct’.
‘We see this as a natural evolution of AI in the enterprise: moving from co-pilots to true collaborators. The advantage is not just automation, but intelligent orchestration where AI agents can analyse processes, identify inefficiencies, recommend or take action and continuously improve over time,’ he adds.
Similarly, José María Alonso, Snowflake’s country manager for Spain and Portugal, believes that ‘the main novelty is that agentic AI is not limited to the simple automation of repetitive tasks, but is capable of making complex decisions and dynamically adapting to constantly changing environments’. And this translates into higher productivity, more informed decision-making, efficient task automation, personalisation and scalability.
Where does it stand?
Agentic AI is currently in the development phase, but it is advancing at a rapid pace. ‘Agentic AI is rapidly moving from proof-of-concept to production. The fundamental models are already here and now the domain-specific capabilities are being built, such as integration with ERP systems, understanding business logic and reasoning with real-time process data,’ says Celonis.
But there is still a lot of work ahead. ‘Key areas of development include improving reasoning capabilities, reducing the error rate, ensuring ethical and unbiased responses, and perfecting multi-step problem-solving skills,’ says Daniel Agudo, head of Technology Business Development at Concentrix Spain.
In addition, Monzonis believes that ‘one of the next steps must be to activate adoption processes’, which entails a cultural transformation, not just a technological one. ‘We have to prepare people so that they can make proper use of these systems and get the most out of them’.
Galfi also points out that next steps ought to be made in three directions. First, trust and governance, ‘so that agentic systems are explainable, controllable and secure’. Secondly, scalability, ‘allowing organisations to deploy and manage fleets of agents across multiple departments’. And finally, in the adoption of feedback loops, ‘creating learning mechanisms so that agents can continuously improve based on results.
In addition, the head of Concentrix emphasises that ‘a key driver for leveraging generative AI systems is data governance’. ‘The better the quality of the data, the better the results in terms of productivity and customer satisfaction,’ he says.
Multiple applications of Agentic AI
In terms of specific applications of this technology, the Concentrix spokesperson highlights ‘autonomous or minimally human-supervised handling of complex customer queries, automated and personalised product recommendations, real-time support, personalised digital marketing, intelligent complaint resolution systems, supply chain optimisation and improved healthcare case management’.
Salesforce also points to some of the new possibilities offered by agentic AI in a number of areas. One of these is the ability to deliver ‘proactive and personalised’ customer service through intelligent agents. ‘Companies can offer more human and responsive conversational experiences. These agents not only respond, but also anticipate needs, automatically escalate cases and execute actions, such as generating refunds or scheduling appointments, without human need,’ he says.
Agentic AI also offers opportunities in context-aware sales automation. ‘Agents take action, not just suggesting next steps, but executing them: sending personalised emails, updating opportunities and proposing closing strategies based on historical data and real-time behaviour,’ the company says.
In addition, new possibilities are inaugurated in the deployment of hyper-personalised marketing actions. ‘Agentic AI allows each customer interaction to be unique and relevant. Agents dynamically generate, test and optimise campaigns, adapting content and channel based on the customer profile and previous interactions,’ it notes.
Salesforce also highlights the technology’s ability to provide internal support and employee productivity. ‘In environments such as Slack, intelligent agents assist employees with contextual responses, task automation and immediate access to knowledge, improving the employee experience and reducing resolution time.
The Celonis spokesperson also notes that ‘agents can be trained for specific, high-impact business workflows, such as invoice processing, customer onboarding or supply chain exceptions, and are already demonstrating tangible productivity improvements’.
Snowflake’s country manager says: ‘Agentic AI applications span a wide range of areas and can help transform the way businesses operate and engage with their customers.
‘In customer service, we can expect to see virtual agents capable of delivering personalised support on a large scale, answering questions, solving problems and proactively anticipating customer needs. In data analytics, agentic AI will make it possible to analyse large volumes of information to identify trends, patterns and hidden opportunities, providing valuable information for strategic decision-making. Process automation will be revolutionised by agents capable of executing repetitive and complex tasks with greater efficiency and accuracy, freeing employees to focus on activities of greater strategic value. Finally, in decision-making, agentic AI will provide accurate and personalised information and recommendations, helping leaders make more informed and strategic decisions based on objective data and analytics,’ he details.
And its results are already tangible. ‘We have seen that it is able to resolve 14% more customer service issues every hour. In addition, it reduces by 90% the time spent on ‘sales prospecting’, i.e. the time salespeople spend identifying and qualifying potential customers,’ he specifies.
Most Benefited Sectors
Among the sectors most active in the adoption of agentic AI, Agudo highlights retail (virtual agents to accompany the entire purchasing process), travel (service recommendations), insurance (appointments and case management), banking (automated trading), healthcare (personalised treatment plans), marketing and logistics (optimised supply chains).
Monzonis also highlights its applications in the public sector (citizen care through mechanisms that allow for the expansion of channels and quality service), industry and supply chain (robotisation and automation of cognitive processes in the manufacturing process, distribution, warehouse, last mile? ), sustainability (optimisation and rationalisation in the use of resources, as well as traceability and access to carbon footprint information), banking and insurance (automation of the contact centre and other core banking processes), retail (intelligent assistants with autonomy in decision-making in different scenarios), education (development of learning experiences adapted to individual needs) or health (improvement of patient care and research into new medicines).
Galfi also underlines its potential in industries with complex and interconnected processes. ‘For example, in the manufacturing industry, AI agents are helping to manage supply chains, quality assurance and production scheduling,’ he says.
But it is also impacting other sectors. In healthcare, he says that ‘AI agents are improving patient flow, reducing administrative burdens and ensuring compliance’. In the public sector, he notes that agentic AI ‘is creating greater transparency and accountability over how agencies are using taxpayer funds’.
In financial services, he notes that companies can use AI agents to optimise loan processing, fraud detection and customer onboarding. And in the retail and consumer goods sector, AI agents can be used to streamline order management, forecast demand and reduce returns.
In addition, Salesforce believes it will aid seasonal industries such as retail and tourism. ‘These companies can double or triple their sales activity at specific times of the year. Thanks to the autonomous agents, they can take on and execute tasks such as resolving complaints, managing item returns or making personalised offers’, the company explains.
Difficulties in adoption…
While there are many benefits to adopting agentic AI, incorporating the technology into organisations is not so straightforward, as it comes with its own set of challenges. For example, Galfi points out that one of the challenges is identifying the problem to be solved. ‘AI agents work well when the problem they need to solve is clearly defined and very limited in scope. Otherwise, they struggle. For most businesses, identifying the right problem to solve will be key to getting real value from AI agents.’
He also believes it can be tricky to write messages that work. ‘As LLMs improve, writing work instructions for AI agents will become easier. But right now it’s a long, iterative process that makes it difficult to create agents. Since the value an agent provides depends largely on the quality of the instructions and configuration settings it receives, agent developers need the right tools to create effective agents at scale,’ he says.
And another tricky aspect is the monitoring of actions and decisions. ‘AI agents act more like humans than other automation systems or software. While this has its advantages, it also means they are easy to fool. They are more likely to give inconsistent answers and forget things, or even hallucinate from time to time. Agents require governance and continuous improvement, so companies must be able to track agents’ actions and decisions and understand their impact on business processes. Agents should also be implemented in accordance with the principles of responsible AI,’ he stresses.
The Capgemini spokesperson also talks about the return on investment and the talent required for deployment, as ‘companies must have the right people with the right knowledge for implementation, as well as the investment decision to carry it out’.
Another sensitive aspect is the predictability and reliability of agentic AI, so efforts must be made to ‘ensure that the actions and responses provided are within an appropriate framework, which must be analysed and for which the boundaries of action of AI agents must be established’.
And agentic AI also poses a challenge in terms of data privacy and security. Monzonis stresses that it is necessary to ‘ensure the protection of personal data and the security of key information, as well as the mechanisms for accessing it’.
In line with this, Salesforce stresses the importance of data quality. ‘AI is never going to be better than the data a company has, so to build trust, it is crucial to ensure that AI systems use accurate and relevant data, maintain privacy, and operate within ethical and legal boundaries. This means implementing robust data governance and oversight, as well as a strong layer of security to protect it.
Alonso also believes that ‘the main obstacle lies in the quality of the underlying data, as the agent’s decisions are based on this information’. ‘The lack of adequate data quality and governance in organisations becomes a major impediment to the successful adoption of intelligent agents,’ he warns.
…And potential risks of use
All of this leads to some potential usage risks. The first is legal risk, ‘related to regulatory compliance (AI Act in Europe), risks of fines or intellectual property rights, data leakage, risk of vulnerabilities, etc.,’ says the Capgemini expert.
Another is the risk of adoption, ‘referring to the transition to a new working model in companies with the use of AI agents, from the point of view of appropriate use and consumption, training, governance or organisation of people’.
And finally, reputational risk, linked to the ‘control of the negative impact that may be caused by an improper or unethical use of AI or other aspects related to the bias and management of the response offered by AI’, he specifies.
Celonis’ director of product management also stresses the importance of these tools “ensuring appropriate levels of security and transparency, to make sure that agents interacting with sensitive systems are tightly governed, and that companies can explain and audit what AI is doing and why”.
He further notes that ‘some of the main concerns are that agents will make decisions outside acceptable limits, show bias or propagate errors if training data or agent logic is flawed’.