Measuring the Sustainability of AI
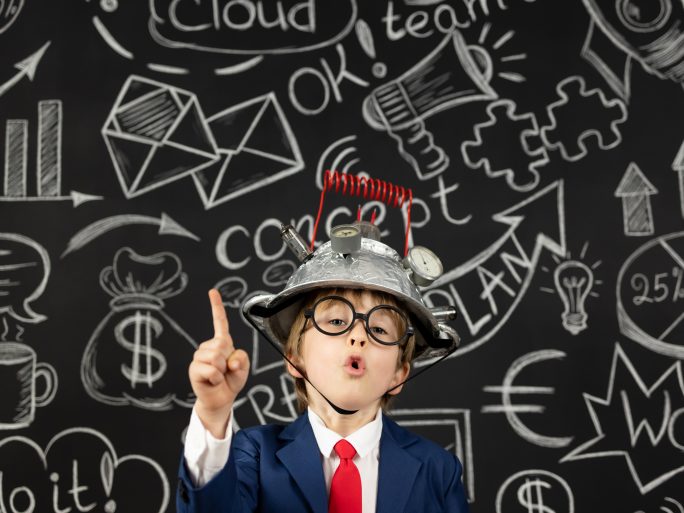
How many resources AI applications require is largely unknown, although a lot of data could have been measured automatically long ago.
Researchers from the Institute for Ecological Economy Research (IÖW) point this out. Together with the non-governmental organisation AlgorithmWatch and the Distributed Artificial Intelligence Laboratory at the Technical University of Berlin, they have spent three years investigating how AI applications can become more sustainable in the “SustAIn” lighthouse project with funding from the German Federal Ministry for the Environment. In their recommendations, they call for the sustainability impact of AI to be measured more closely throughout its life cycle and for the development and use of AI systems to be regulated.
Comparing emissions over the entire life cycle
In their report “Taking (policy) action to enhance the sustainability of AI systems“, the researchers show how energy consumption can be measured during the development and training phases
” In particular, the providers of large language models, known as LLMs, often only state the direct energy consumption and emissions for a training cycle,” explains AI expert Friederike Rohde from the IÖW. “This leaves the picture incomplete. If hardware production and operating energy are also taken into account, the emissions value can quickly double. In addition, continuous emissions occur during the application of the model. Indicators suggest that these could be immense.”
According to the experts, although the EU AI regulation lists environmental aspects for the first time, these are not enough. “We are pleased that the AI Act is taking the first steps towards making the environmental risks of artificial intelligence comprehensible. Measuring the energy and resource consumption of AI is possible and urgently needed, as we have shown in our project,” says Kilian Vieth-Ditlmann from AlgorithmWatch. “But further approaches are needed to regulate the environmental impact. For example, measurement and reporting standards should also be developed for the AI utilisation phase, for example by AI providers defining various standard usage scenarios before the market launch.”
Creating transparency about energy consumption
In their study, the researchers show which data should be collected with regard to energy consumption during system development and model training. Metrics such as power usage effectiveness, which make transparent how much energy a data centre uses for data processing in relation to its total energy consumption, could help here. This parameter can be used to compare the energy efficiency of data centres.
A case study on AI in personalised online advertising also showed how relevant it is to monitor energy and resource consumption. “Either energy consumption is not measured at all or the data is held by large IT companies such as Google, Facebook or Amazon, which do not make it transparent,” explains Gesa Marken from the IÖW. “We are calling for the introduction of legal obligations to measure and publish such data.”
Consider the economic and social impact of AI
The researchers point out that the strong market concentration of the AI industry leads to global distribution inequalities. Therefore, the problematic trends in AI development from an economic and social perspective should also be taken into account. “In order to tackle the problems of market concentration, the European Union has regulated the large online platforms in the tech industry with the Digital Markets Act (DMA). This could be a model for how market concentration in the AI sector could be reduced,” says digital expert Josephin Wagner from the IÖW. “Further political initiatives should now follow suit to ensure the sustainable development and use of AI systems. We recommend requirements for data governance, legislation for the supply chain and a strong eco-design regulation.”