Protect Sensitive Machine Data
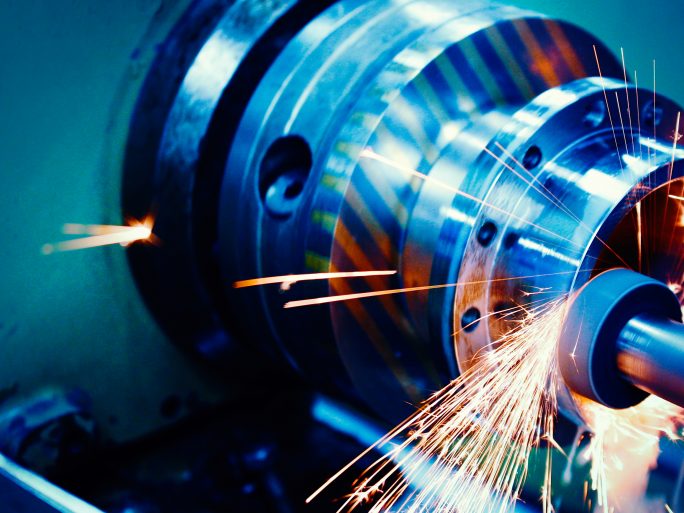
Ensure the security of sensitive machine data with technologies from data protection.
To harness the potential of machine data for data-driven innovation, it must be aggregated and analysed across companies. In this way, operators of data infrastructures can offer collaboratively trained machine learning applications with which, on the one hand, users can improve the efficiency and effectiveness of their production plants through predictive condition monitoring and, on the other hand, machine manufacturers can systematically identify optimisation potential in their products.
On the other hand, however, there is the concern that the provision of data could result in the loss of intellectual property and thus competitive disadvantages. For this reason, there has hardly been any data exchange between companies, which is a prerequisite for data-driven innovations.
Machine learning models for predictive maintenance
In the research project “Secure Collaborative Exploitation of Machine Tool Data with the Help of Privacy Enhancing Technologies” (MINERVA), the Fraunhofer Institute for Applied and Integrated Security AISEC is working with partners to develop technologies for a data infrastructure that uses technologies from data protection to ensure the security of sensitive machine data. The research network includes the Institute for Machine Tools and Industrial Management (iwb) at the Technical University of Munich, Hufschmied Zerspanungssysteme and Siemens. Using anonymised data, the partners train suitable machine learning models for the predictive condition monitoring of machine tools, which then function without disclosing sensitive data.
Protecting sensitive data
“Privacy enhancing technologies have so far been used for the protection of personal data, for example to evaluate patient information anonymously (e.g. with “differential privacy”). The project partners are now transferring the principles of this approach to data from the machine tool industry in order to protect the intellectual property of the data providers.
“If machine learning algorithms are trained in the cloud with cross-operator data, it would be possible to understand, for example, what kind of workpiece was produced (product geometry), how the machine tool was utilised, how much energy was consumed during production and at what speed production took place. From the user’s point of view, this is very sensitive information, and we are developing solutions to protect it in MINERVA,” says Bartol Filipovic, MINERVA project manager at Fraunhofer AISEC, describing the added value of the research approach.
In addition to “differential privacy”, “trusted execution environments” for the realisation of a protected, attested area in the cloud or “federated learning”, in which not the data itself but only the ML models already trained in the edge migrate to the cloud, are among the technologies from data protection that are used in MINERVA.