Salesforce: “Accuracy is the Most Important Thing When Applying AI in a Business Context”.
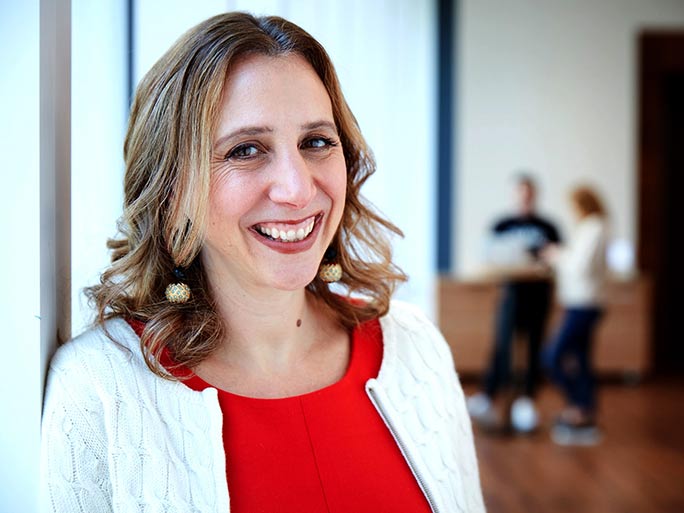
Paula Goldman, Chief Ethical and Humane Use Officer at Salesforce, explains how they deal with the toxicity generated by Artificial Intelligence.
During Salesforce TrailblazerDX, the company’s developer event to announce the launch of Einstein GPT, Silicon had the opportunity to interview Paula Goldman, Chief Ethical and Humane Use Officer at Salesforce.
To start our conversation, Paula said that in 2018 they defined the principles that would govern Salesforce’s work on artificial intelligence in general. Once Einstein GPT started to become real for the company they defined the principles applied to OpenAI artificial intelligence focused on customer services.
– Let’s talk about Einstein GPT’s ethical principles. How are they working? There are different regions in the world and I guess it’s difficult to adapt them everywhere. How are you doing so?
Yes, absolutely. Let’s go back on the principles a little bit. They are accuracy, safety, honesty, empowerment and sustainability. As you can see they are overarching principles. So, if we think particularly accuracy is the most important thing when applying AI in a business context, because you have to make sure that if the AI is making a recommendation for a prompt, for a customer chat or a sales-focused email, that it’s not making up facts. And that’s true across cultures, across geographies. We want to make sure that we generate results that are actually based on our customers’ data models and, if we don’t know the answer, that the AI has the ability to say “I don’t know” or “this is not a relevant question”. However, there are places where the answer may vary depending on geography and it has to do with bias and toxicity. That is, when we think about how different demographic groups should be represented within the data, how we label words as toxic content, it requires a very culturally specific approach. It requires a lot of care across cultures. And that’s something we’re paying a lot of attention to.
– When you talk about AI behaviour sometimes generating inappropriate content, how do you deal with that?
Neither bias nor toxicity is a new problem for generative AI. Generally speaking, AI is only as good as the data you give it and you have to make sure that the datasets are representative, that there is no unintentional bias in the data, label it as toxicity for these types of problems. For generative AI, especially when we talk about these extremely large base models, there are increasingly sophisticated solutions to these problems that you mention. And it has to do with the size of these models. And in some cases, they are open errors. So, we can send you some of these papers, but there are a number of research papers that talk about evaluation and basically taking the time to see if you can break the model and then fix it iteratively. That’s what a number of generative AI models have been trained on. And it’s a methodology that we also use. The reason I give you that example is to say that it gets more and more sophisticated when we think about the more expansive uses of generative AI, and then you have to pay additional attention to that kind of thing. And there is an emerging state of the art in those techniques.
– Do you have AI ready for impertinent customers? If you have an impertinent customer who is typing in inappropriate language, is the AI educated to respond in those kinds of cases?
Well, that’s an interesting question. The way it would work more generally would be, for example, if we have an AI set up to help a customer service agent and someone asks an off-topic or even rude question, I think the AI would most likely say they don’t know or it’s not within the boundaries and the content they’re commenting on. It may also be possible to suggest phrases to de-escalate a conversation. But I always think the AI will stay within the boundaries to make sure those conversations are appropriate and healthy, and based on real business facts and data.
– And when you find a bug in the AI behaviour, do you report it to OpenAI? How do you work in both companies?
First of all I will say that the way we are designing Einstein GPT is to give our customers choices in different models and start by understanding that you can start with a starting point. What are the properties of these different models around these issues, around biases, toxicity, etc.? And those are published things that we can talk about and then give our clients a lot of choice. We can also educate them and inform them about how to use this generative Ia in a responsible way. Secondly, we’re doing a lot of testing ourselves, as we create these products and design these integrations, some of that feedback is fed back into our own design. And I would say the vast majority of that feedback is incorporated into our own design because we talk about how AI is grounded in our customers’ data. So, we could ground the AI in 100 knowledge articles from a customer and then train them to say that if a question wasn’t found, the answer wasn’t found in those knowledge articles, they could say “I don’t know”. The vast majority of the work is going to be making sure that that cycle that we’re building works, and I think we have a very collaborative relationship as well with these different associations in providing feedback to them. We’re working with them on responsible approaches as they continue to evolve. We’re working with them as we continue to iterate on these features of the products themselves. But I think a lot of the feedback we’re going to have on the “human loop” that we’re talking about, the pilot processes and the safeguards, the feedback we’re looking for from our customers is on how we’re designing the product itself.
– Will the content that Einstein GPT generates be identified as having been generated by artificial intelligence?
Yes. What I can say is that if there is something that is only AI-generated, it will be marked as such. I don’t know if it will be a particular watermark, but rather, if you’re just interacting with an AI, it will obviously be clear to you that you’re interacting with an AI and the way we design products, for example, if the AI suggests or drafts an email, there must be a human involved to approve, change, edit and send. The AI will not send it autonomously.
– How can AI know what information is private to the company and what information it can show to the customer?
That’s a critical question that has a lot to do with the design and testing that we’re creating. And, again, going back to the example of anchoring the data and making sure that we’re clear on the data set that we want it to refer to. I would say that both the partners we are working with and our own teams are also doing a lot of security and privacy testing to make sure that the model cannot be broken and make it reveal private and secure information. That’s something we emphasise in the principles around responsible generative AI, and it’s something we’re working very hard on.
– Can you tell me some cases where your AI failed, generating inappropriate content or something like that?
For example, we have an existing chatbot that works in a service capacity and we do a lot of testing before we release something to the market. We noticed when we were working on the chat and looking at the training data that we needed it to be more representative. In the US context, but how do you bring in different vernaculars, different ways of speaking English? There is something called African American vernacular. There are a number of different informal ways of speaking that can influence the success of an interaction with a chatbot. And you want to make sure that those are represented, that that data is representative of the customers that they serve in the end, which is a broad group. And so we work with that team to make sure that we augment the training data to be as representative as possible. There are a lot of examples like that where we say, “Hey, we have a set of standards on AI that we’re launching and we’re going to catch an issue before it hits the market to make sure it’s right.”
– To conclude the interview, what is the next step for Einstein GPT?
I’m very excited about where we are around generative artificial intelligence, not only because I think we’ve thought carefully about how to use it in a business context and how to carefully design to make sure that the data that you put in is good and that the answer you get back is accurate, useful and reliable. But I’m also very excited about the work we’re doing on the ethics front because we’re not starting from scratch. At Salesforce, we’ve been working on this for almost five years. We’re working with a lot of experts outside the company. We’re working with civil society groups and applying everything we’ve learned going forward. And I feel like this is the moment where we talk about technology ethics. This is what it was created for. It was created for a time when we are taking a technology that is still in its infancy, where we don’t yet know all the use cases and where it will be applied. But we are thinking and applying foresight. And for me, that’s the most exciting thing, is this stage where we are really testing these principles and finding their outcomes.